Cost-Effective Affordable AI for Breast Lesion Detection with Ultra-sensitive Optical Sensing
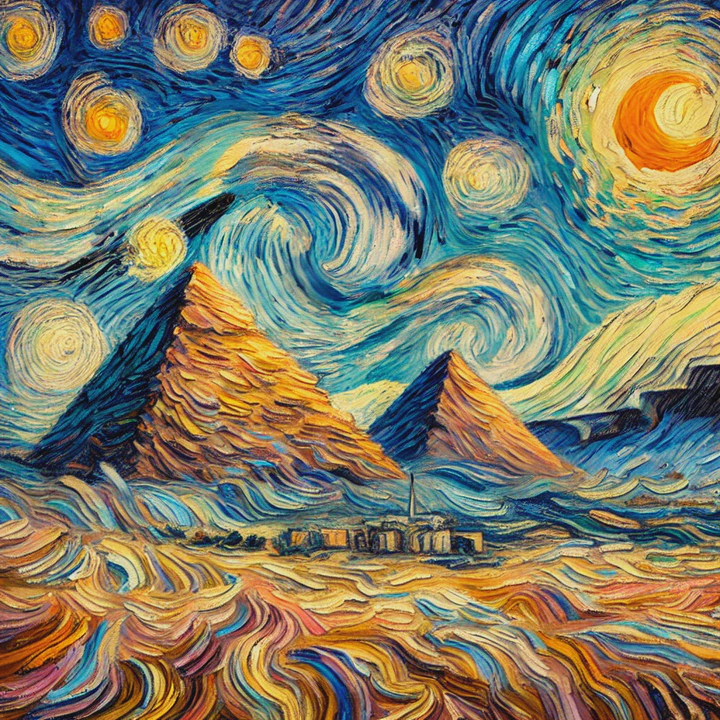
Breast cancer is a global health crisis and a leading cause of cancer-related mortality among women, with over 2.3 million new cases and approximately 685,000 deaths reported in 2020. Early and accurate detection is crucial for successful treatment outcomes, yet traditional diagnostic methods like mammography, biopsy, and digital pathology are expensive, invasive, and require highly specialized infrastructure, making them inaccessible in low-resource settings. Thus, there is an urgent need for diagnostic tools that are both affordable and effective.
Current Challenges: While micro-optical resonators offer high sensitivity, their translation into clinical settings requires solutions for stability, compatibility with existing medical equipment, and real-time data handling. Moreover, accurately distinguishing between benign and malignant tissue using ML remains challenging, requiring model fine-tuning and rigorous evaluation to ensure reliability comparable to digital pathology.
This project aims to develop a novel diagnostic approach that combines biosensor technology with advanced Machine Learning (ML) and Deep Learning algorithms for breast lesion detection and grading. By leveraging cost-effective biosensor-based detection, we seek to deliver comparable accuracy to digital pathology methods while minimizing expense and resource requirements. This approach not only holds promise for affordable diagnostics but also addresses the unmet needs of low-resource settings, contributing to broader health equity.
Project goals
- To develop a novel, non-invasive, biosensor-based diagnostic tool for breast lesion detection and grading that is accessible and cost-effective.
- To integrate advanced ML and Deep Learning algorithms specifically optimized to analyze data from biosensors, providing a high-accuracy, low-cost alternative to digital pathology.
- To rigorously compare the efficacy of this biosensor-based approach with digital pathology, aiming to validate its reliability in detecting and grading breast lesions.
- To establish a collaborative framework between the GUC ARAtronics Research Center at the German University in Cairo (GUC) and Albarqouni Lab at the University Hospital Bonn, University of Bonn, combining their strengths in bio-optical sensors, ML, and medical imaging, and advancing solutions for low-resource clinical environments.
Activities:
- Kickoff Meeting at UKBonn
- Summer School on Affordable AI at GUC
- Short Research Stays at UKBonn
- Workshop on Affordable AI at the GUC
Partners:
- Assoc. Prof. Amir Ali, Dr. Sally Ibrahim, and Dr. Shereen Afifi from German University in Cairo, Egypt
- Prof. Shadi Albarqouni from University Hospital Bonn | Universiy of Bonn | Helmholtz AI, Germany
Funding:
The project is financed by the German Academic Exchange Service (DAAD) with funds from the German Federal Ministry of Education and Research (BMBF).