Modelling Uncertainty in Deep Learning for Medical Applications
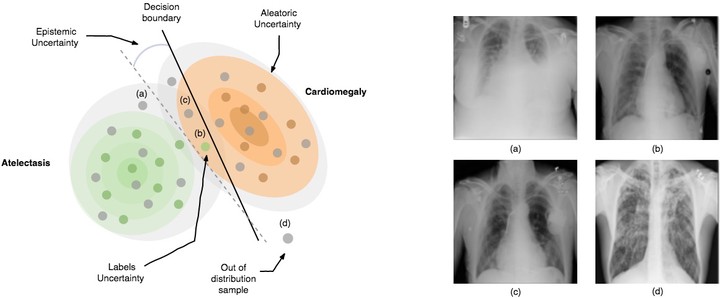
Deep Learning has emerged as a leading technology for accomplishing many challenging tasks showing outstanding performance in a broad range of applications in computer vision and medical applications. Despite its success and merit in recent state-of-the-art methods, DL tools still lack in robustness hindering its adoption in medical applications. Modeling uncertainty, through Bayesian Inference and Monte-Carlo dropout, has been successfully introduced to computer vision for better understanding the underlying deep learning models. In this proposal, we investigate modeling the uncertainty for medical applications given the well-known challenges in medical image analysis, namely severe class-imbalance, few amounts of labeled data, domain shift, and noisy annotations.
Collaboration:
Prof. Ender Konukoglu, Department of Information Technology and Electrical Engineerng, ETH Zurich.
Prof. Daniel Rueckert, Department of Computing, Imperial College London
Prof. Nassir Navab, Faculty of Informatics, Technical University of Munich
Funding:
This project is supported by the PRIME programme of the German Academic Exchange Service (DAAD) with funds from the German Federal Ministry of Education and Research (BMBF).