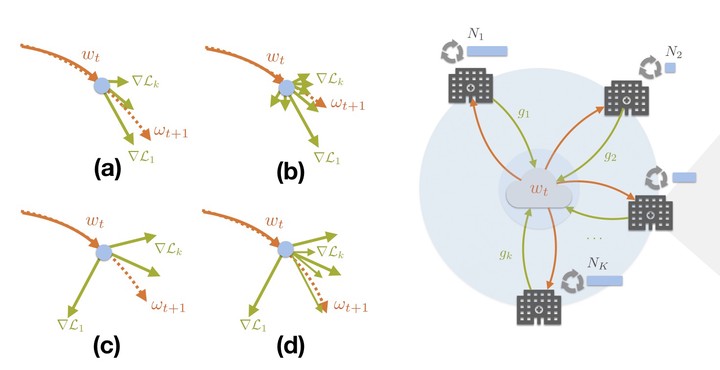
Deep Learning (DL) has emerged as a leading technology for accomplishing many challenging tasks showing outstanding performance in a broad range of computer vision and medical applications. However, this success comes at the cost of collecting and processing a massive amount of data, which often are not accessible, in Healthcare, due to privacy issues. Federated Learning (FL) has been recently introduced to allow training DL models without sharing the data. Instead, DL models at local hubs, i.e. hospitals, share only the trained parameters with a centralized DL model, which is, in return, responsible for updating the local DL models as well.
Our golas in this project is to develop novel models and algorithms for a ground-breaking new generation of deep FL, which can distill the knowledge from local hubs, i.e. hospitals, and edges, i.e. wearable devices, to provide personalized healthcare services.
The principal challenges, to overcome, concern the nature of medical data, namely data heterogeneity; severe class-imbalance, few amounts of annotated data, inter-/intra-scanners variability (domain shift), inter-/intra-observer variability (noisy annotations), system heterogeneity, and privacy issues (see the example below).
Collaboration:
Funding:
- Helmholtz Young Investigator Group (1.8 Million Euros)
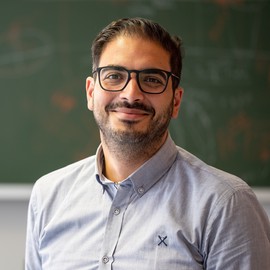
Shadi Albarqouni
Professor of Computational Medical Imaging Research at University of Bonn | AI Young Investigator Group Leader at Helmholtz AI | Affiliate Scientist at Technical University of Munich
Related
Publications
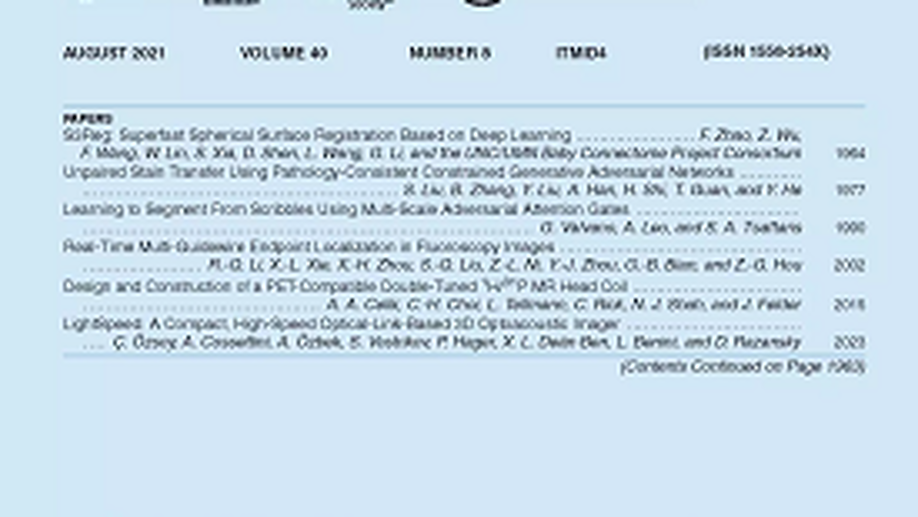
Guest Editorial Special Issue on Federated Learning for Medical Imaging: Enabling Collaborative Development of Robust AI Models
Federated Learning (FL) could solve the challenges of training AI models on large datasets for medical imaging due to data privacy and ownership concerns by allowing collaborative training without the need for sharing raw data. This Special Issue on Federated Learning for Medical Imaging features papers covering FL-related topics and discussing their implications for healthcare and medical imaging. The included articles focus on a broad range of federated scenarios and applications, such as semi-supervised and self-supervised learning, histopathology, image reconstruction, graph neural networks, privacy preservation, active learning, data auditing, multi-task learning, personalization, and swarm learning. The importance of training unbiased, privacy-preserving, and generalizable AI models that have the potential to be translated into clinical practice increases the need for collaborative training techniques such as FL. The articles included in this Special Issue have moved the needle markedly forward in this regard.
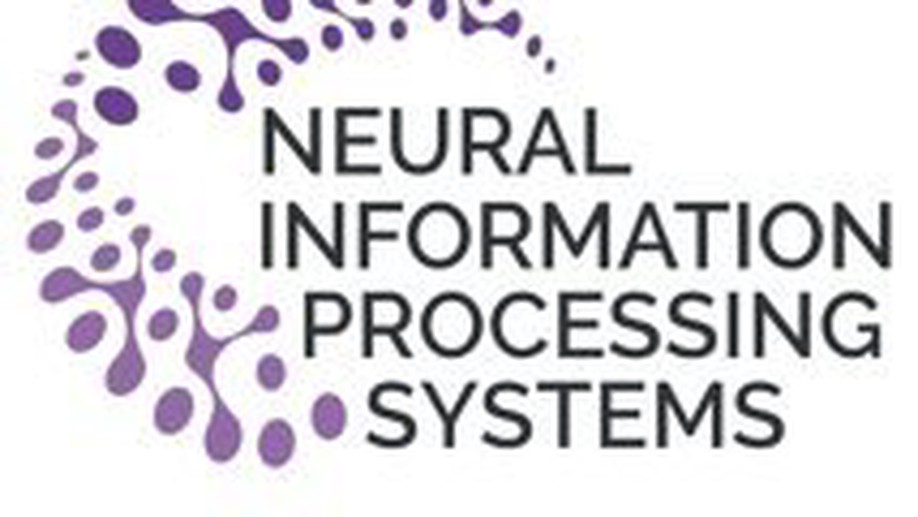
FLamby: Datasets and Benchmarks for Cross-Silo Federated Learning in Realistic Healthcare Settings
Federated Learning (FL) is a novel approach enabling several clients holding sensitive data to collaboratively train machine learning models, without centralizing data. The cross-silo FL setting corresponds to the case of few (–) reliable clients, each holding medium to large datasets, and is typically found in applications such as healthcare, finance, or industry. While previous works have proposed representative datasets for cross-device FL, few realistic healthcare cross-silo FL datasets exist, thereby slowing algorithmic research in this critical application. In this work, we propose a novel cross-silo dataset suite focused on healthcare, FLamby (Federated Learning AMple Benchmark of Your cross-silo strategies), to bridge the gap between theory and practice of cross-silo FL. FLamby encompasses 7 healthcare datasets with natural splits, covering multiple tasks, modalities, and data volumes, each accompanied with baseline training code. As an illustration, we additionally benchmark standard FL algorithms on all datasets. Our flexible and modular suite allows researchers to easily download datasets, reproduce results and re-use the different components for their research.
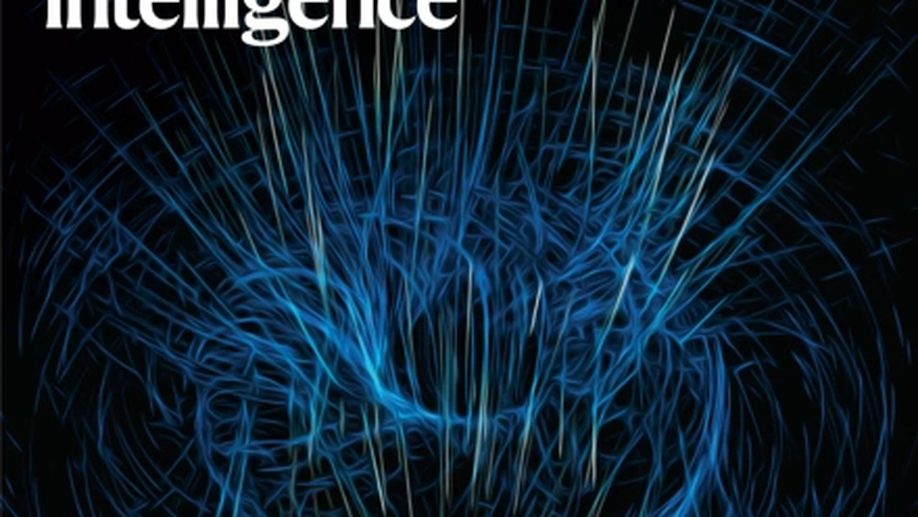
Federated disentangled representation learning for unsupervised brain anomaly detection
With the advent of deep learning and increasing use of brain MRIs, a great amount of interest has arisen in automated anomaly segmentation to improve clinical workflows; however, it is time-consuming and expensive to curate medical imaging. Moreover, data are often scattered across many institutions, with privacy regulations hampering its use. Here we present FedDis to collaboratively train an unsupervised deep convolutional autoencoder on 1,532 healthy magnetic resonance scans from four different institutions, and evaluate its performance in identifying pathologies such as multiple sclerosis, vascular lesions, and low- and high-grade tumours/glioblastoma on a total of 538 volumes from six different institutions. To mitigate the statistical heterogeneity among different institutions, we disentangle the parameter space into global (shape) and local (appearance). Four institutes jointly train shape parameters to model healthy brain anatomical structures. Every institute trains appearance parameters locally to allow for client-specific personalization of the global domain-invariant features. We have shown that our collaborative approach, FedDis, improves anomaly segmentation results by 99.74% for multiple sclerosis, 83.33% for vascular lesions and 40.45% for tumours over locally trained models without the need for annotations or sharing of private local data. We found out that FedDis is especially beneficial for institutes that share both healthy and anomaly data, improving their local model performance by up to 227% for multiple sclerosis lesions and 77% for brain tumours.
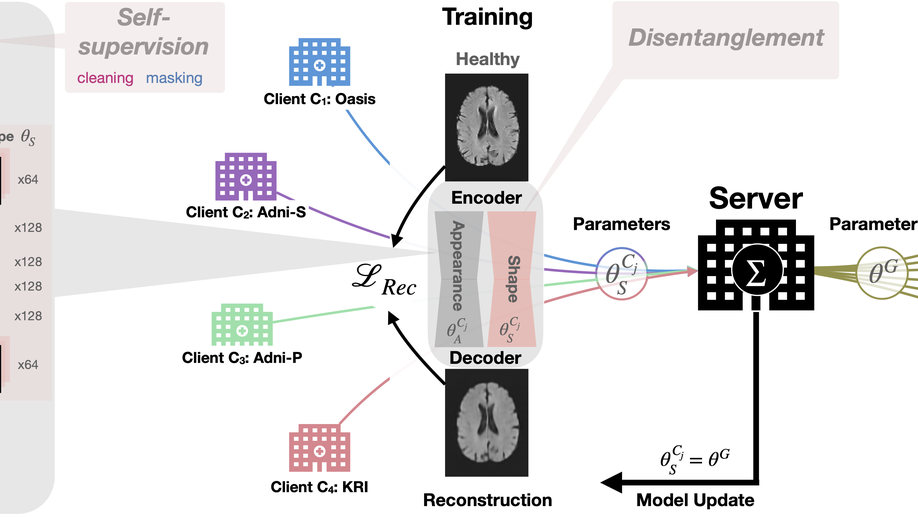
Federated Disentangled Representation Learning for Unsupervised Brain Anomaly Detection
Recent advances in Deep Learning (DL) and the increased use of brain MRI have provided a great opportunity and interest in automated anomaly segmentation to support human interpretation and improve clinical workflow. However, medical imaging must be curated by trained clinicians, which is time-consuming and expensive. Further, data is often scattered across multiple institutions, with privacy regulations limiting its access. Here, we present FedDis (Federated Disentangled representation learning for unsupervised brain pathology segmentation) to collaboratively train an unsupervised deep convolutional neural network on 1532 healthy MR scans from four different institutions, and evaluate its performance in identifying abnormal brain MRIs including multiple sclerosis (MS), vascular lesions, low-grade tumors (LGG), and high-grade tumors/glioblastoma (HGG/GB) on a total of ~538 scans from 6 different institutions and datasets. To mitigate the statistical heterogeneity between the different institutes, we disentangle the parameter space into global, i.e., shape and local, i.e., appearance. We train the shape parameters jointly from four institutes to learn a global model of the healthy anatomical brain structure. The appearance parameters are trained locally on every institute and allow for personalization of the global domain-invariant features with client-specific information, such as scanner or acquisition parameter. We have shown that our collaborative approach, FedDis, improves anomaly segmentation results by 99.74% for MS, 83.33% for vascular lesions,and 40.45% for tumors over locally trained models without the need for annotations or sharing private local data. We found out that FedDis is especially beneficial for clients that share both healthy and anomaly data coming from the same institute, improving their local anomaly detection performance by up to 227% for MS lesions and 77% for brain tumors.
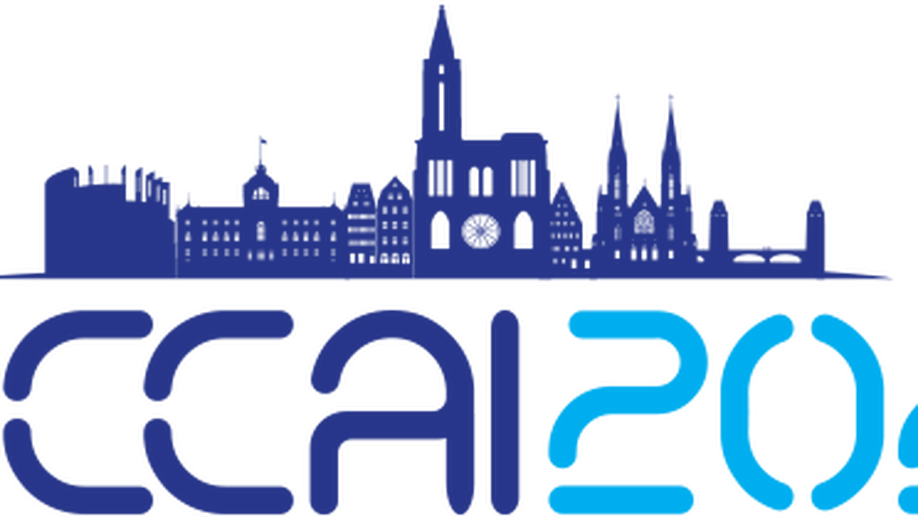
FedPerl: Semi-Supervised Peer Learning for Skin Lesion Classification
Skin cancer is one of the most deadly cancers worldwide. Yet, it can be reduced by early detection. Recent deep-learning methods have shown a dermatologist-level performance in skin cancer classification. Yet, this success demands a large amount of centralized data, which is oftentimes not available. Federated learning has been recently introduced to train machine learning models in a privacy-preserved distributed fashion demanding annotated data at the clients, which is usually expensive and not available, especially in the medical field. To this end, we propose FedPerl, a semi-supervised federated learning method that utilizes peer learning from social sciences and ensemble averaging from committee machines to build communities and encourage its members to learn from each other such that they produce more accurate pseudo labels. We also propose the peer anonymization (PA) technique as a core component of FedPerl. PA preserves privacy and reduces the communication cost while maintaining the performance without additional complexity. We validated our method on 38,000 skin lesion images collected from 4 publicly available datasets. FedPerl achieves superior performance over the baselines and state-of-the-art SSFL by 15.8%, and 1.8% respectively.
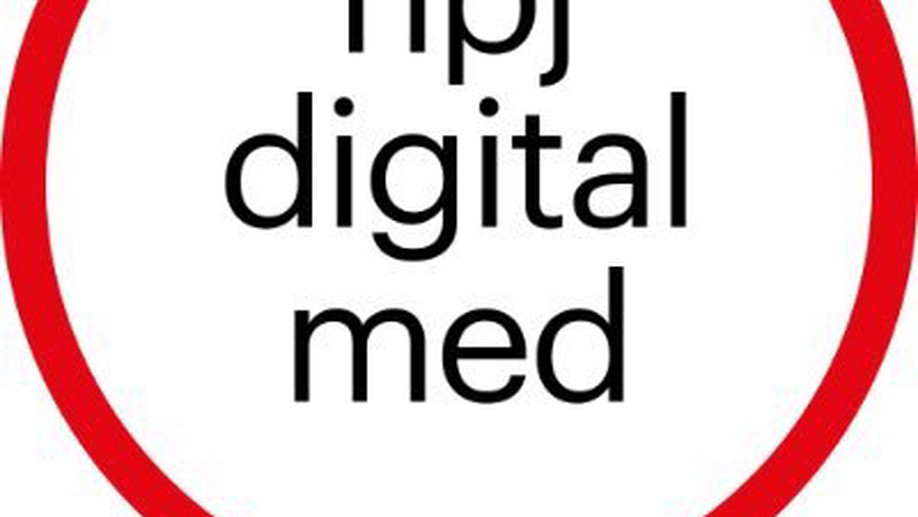
The Future of Digital Health with Federated Learning
Data-driven Machine Learning has emerged as a promising approach for building accurate and robust statistical models from medical data, which is collected in huge volumes by modern healthcare systems. Existing medical data is not fully exploited by ML primarily because it sits in data silos and privacy concerns restrict access to this data. However, without access to sufficient data, ML will be prevented from reaching its full potential and, ultimately, from making the transition from research to clinical practice. This paper considers key factors contributing to this issue, explores how Federated Learning (FL) may provide a solution for the future of digital health and highlights the challenges and considerations that need to be addressed.