Seminar: Artificial Intelligence in Radiology: Applications and Research
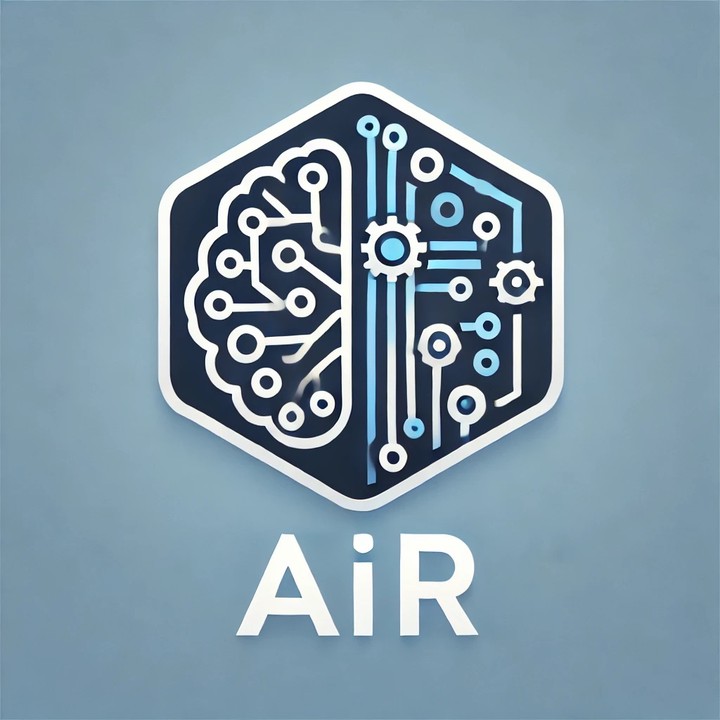
Seminar (2 SWS): Artificial Intelligence in Radiology: Applications and Research (Artifizielle Intelligenz in der Radiologie: Anwendungen und Forschung)
Lecturers: Prof. Dr. Shadi Albarqouni
Tutors: Dr. Elodie Germani, David Gaviria, Yiheneg Xiong
Time: November 15 – Jan 31 (every Friday, 11:30 AM - 1:00 PM 16:00 - 17:30)
Format: Online (Zoom Meeting) with potential face-to-face meetings, if participants interested! Link was provided via email.
Language: English
eCampus: Link to eCampus
Description
The Computational Imaging Research (Albarqouni Lab) at the Clinic of Diagnostic and Interventional Radiology at the University Hospital Bonn is pleased to present a seminar on Artificial Intelligence in Radiology: Applications and Research. This cutting-edge seminar, spanning two semester hours per week (2 SWS), is specially curated for medical students to explore the transformative role of deep learning in medical imaging, with a focus on tasks such as classification, detection, segmentation, reconstruction, tracking, and disease progression.
Course Objectives
Upon completing this seminar, students will be able to:
- Grasp deep learning concepts and their specific applications in medical imaging.
- Analyze advanced image analysis tasks, including classification, detection, segmentation, and disease progression modeling.
- Evaluate ethical and regulatory considerations in the deployment of AI within healthcare.
- Discuss the future potential of AI-driven solutions in personalized medicine and patient care.
Target Audience
This seminar is designed for medical students at the Faculty of Medicine, University Hospital Bonn, who are keen on leveraging AI technologies to enhance their medical practice, specifically within diagnostic imaging.
Prerequisites and Desired Knowledge
- Prerequisites: Familiarity with fundamental medical imaging techniques and an interest in AI applications.
- Desired: Understanding of medical diagnostics and clinical data interpretation.
Seminar Topics and Schedule (Tentative)
Date | Topic | Description | Presenter | Materials |
---|---|---|---|---|
Nov 22 | Deep Learning Foundations | Introduction to deep learning concepts specific to medical imaging. | Meskó, Bertalan, and Marton Görög. “A short guide for medical professionals in the era of artificial intelligence.” NPJ digital medicine 3.1 (2020): 126. (PDF} | |
Nov 29 | Detection and Classification in Medical Imaging | Exploring detection and classification techniques in diagnosing diseases from medical images. | David Gaviria | Litjens, Geert, et al. “A survey on deep learning in medical image analysis.” Medical image analysis 42 (2017): 60-88. (PDF), Chen, Xuxin, et al. “Recent advances and clinical applications of deep learning in medical image analysis.” Medical image analysis 79 (2022): 102444. (PDF), |
Dec 6 | Segmentation | Deep learning methods for segmenting key structures in medical images. | Yiheneg Xiong | Tajbakhsh, Nima, et al. “Embracing imperfect datasets: A review of deep learning solutions for medical image segmentation.” Medical image analysis 63 (2020):101693. (PDF) |
Dec 13 | Causality and Bias | Causality and Bias in deep learning with medical imaging. | Dr. Elodie Germani | Castro, Daniel C., Ian Walker, and Ben Glocker. “Causality matters in medical imaging.” Nature Communications 11.1 (2020): 3673. (PDF), Jones, Charles, et al. “A causal perspective on dataset bias in machine learning for medical imaging.” Nature Machine Intelligence 6.2 (2024): 138-146 (PDF) |
Dec 20 | Moved to the next year due to holiday season | |||
Jan 10 | Q&A Session | Students should prepare questions for their assigned papers and discuss them with the tutors | ||
Jan 17 | Uncertainity Quantification | Quanitifying Uncertainty in Deep Learning Models | David Gaviria | Huang, Ling, et al. “A review of uncertainty quantification in medical image analysis: probabilistic and non-probabilistic methods.” Medical Image Analysis (2024): 103223. (PDF) |
Jan 24 | Student Paper Presentations (1) | Student presentations. | ||
Jan 31 | Student Paper Presentations (2) and Course Wrap-Up | Wrap-up, feedback, and discussion on future AI applications in medical practice. |
Learning Format
One of the seminar’s unique teaching concepts is “Teach It to Yourself.” Participants (limited to 20 partisipants) will present selected chapter and papers, engage in discussions, and critically analyze case studies on deep learning applications in medical imaging.
Registration (closed)
Interested in this seminar, please register via email to Dr. Elodie Germani and Prof. Dr. Shadi Albarqouni
References
- [1] Litjens, Geert, et al. “A survey on deep learning in medical image analysis.” Medical image analysis 42 (2017): 60-88. (PDF)
- [2] Shen, Dinggang, Guorong Wu, and Heung-Il Suk. “Deep learning in medical image analysis.” Annual review of biomedical engineering 19.1 (2017): 221-248.
- [3] Chen, Xuxin, et al. “Recent advances and clinical applications of deep learning in medical image analysis.” Medical image analysis 79 (2022): 102444. (PDF)
- [4] Tajbakhsh, Nima, et al. “Embracing imperfect datasets: A review of deep learning solutions for medical image segmentation.” Medical image analysis 63 (2020):101693. (PDF)
- [5] Wang, Ge, Jong Chul Ye, and Bruno De Man. “Deep learning for tomographic image reconstruction.” Nature machine intelligence 2.12 (2020): 737-748. (PDF)
- [6] Ben Yedder, Hanene, Ben Cardoen, and Ghassan Hamarneh. “Deep learning for biomedical image reconstruction: A survey.” Artificial intelligence review 54.1 (2021): 215-251. (PDF)
- [7] Huang, Ling, et al. “A review of uncertainty quantification in medical image analysis: probabilistic and non-probabilistic methods.” Medical Image Analysis (2024): 103223. (PDF)
- [8] Castro, Daniel C., Ian Walker, and Ben Glocker. “Causality matters in medical imaging.” Nature Communications 11.1 (2020): 3673. (PDF)
- [9] Jones, Charles, et al. “A causal perspective on dataset bias in machine learning for medical imaging.” Nature Machine Intelligence 6.2 (2024): 138-146. (PDF)
- [10] Thirunavukarasu, Arun James, et al. “Large language models in medicine.” Nature medicine 29.8 (2023): 1930-1940. (PDF)
- [11] Zhang, Jingyi, et al. “Vision-language models for vision tasks: A survey.” IEEE Transactions on Pattern Analysis and Machine Intelligence (2024). (PDF)