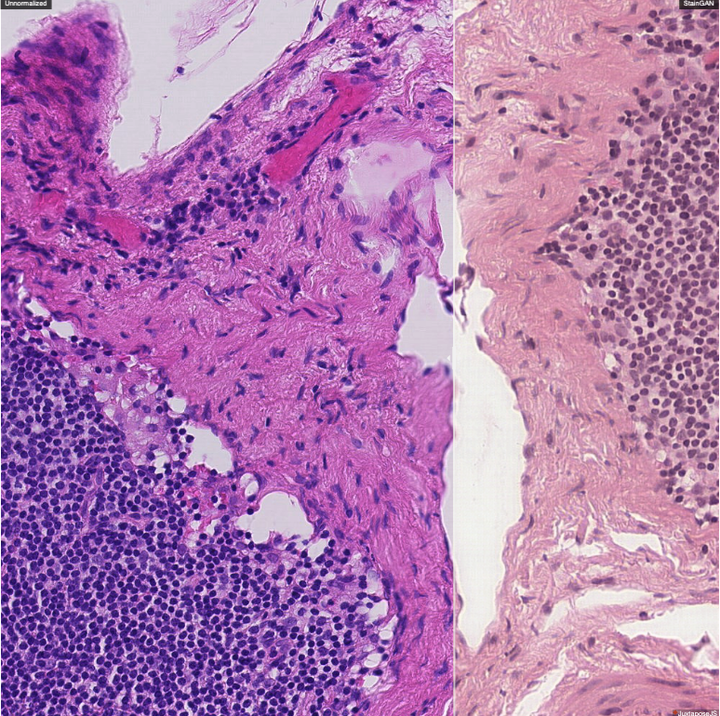
To build domain-agnostic models that are generalizable to a different domain, i.e., scanners, we have investigated three directions; First, Style Transfer, where the style/color of the source domain is transferred to match the target one. Such style transfer is performed in the high-dimensional image space using adversarial learning, as shown in our papers on Histology Imaging (Lahiani et al. 2019a, Lahiani et al. 2019b, Shaban et al. 2019). Second, Domain Adaptation, where the distance between the features of the source and target domains are minimized. Such distance can be optimized in a supervised fashion, i.e., class aware, using angular cosine distance as shown in our paper on MS Lesion Segmentation in MR Imaging (Baur et al. 2017), or in an unsupervised way, i.e., class agnostic, using adversarial learning as explained in our article on Left atrium Segmentation in Ultrasound Imaging (Degel et al. 2018). Yet, another exciting direction that has been recently investigated in our paper (Lahiani et al. 2019c) is to disentangle the feature that is responsible for the style and color from the one responsible for the semantics.
Collaboration:
- Eldad Klaiman, Roche Diagnostics GmbH
- Georg Schummers and Matthias Friedrichs, TOMTEC Imaging Systems GmbH
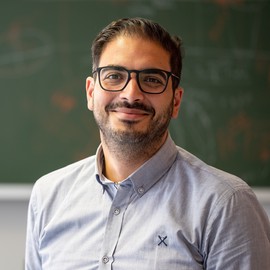
Shadi Albarqouni
Professor of Computational Medical Imaging Research at University of Bonn | AI Young Investigator Group Leader at Helmholtz AI | Affiliate Scientist at Technical University of Munich
Related
- Staingan: Stain style transfer for digital histological images
- Seamless Virtual Whole Slide Image Synthesis and Validation Using Perceptual Embedding Consistency
- Perceptual Embedding Consistency for Seamless Reconstruction of Tilewise Style Transfer
- Learning interpretable disentangled representations using adversarial vaes
- Virtualization of tissue staining in digital pathology using an unsupervised deep learning approach
Publications
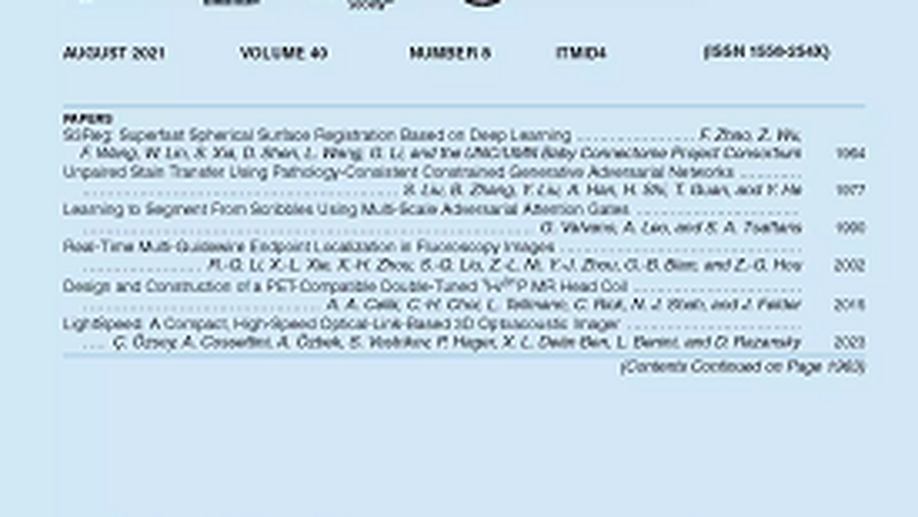
Digital staining of white blood cells with confidence estimation
Chemical staining of the blood smears is one of the crucial components of blood analysis. It is an expensive, lengthy and sensitive process, often prone to produce slight variations in colour and seen structures due to a lack of unified protocols across laboratories. Even though the current developments in deep generative modeling offer an opportunity to replace the chemical process with a digital one, there are specific safety-ensuring requirements due to the severe consequences of mistakes in a medical setting. Therefore digital staining system would profit from an additional confidence estimation quantifying the quality of the digitally stained white blood cell. To this aim, during the staining generation, we disentangle the latent space of the Generative Adversarial Network, obtaining separate representation s of the white blood cell and the staining. We estimate the generated image’s confidence of white blood cell structure and staining quality by corrupting these representations with noise and quantifying the information retained between multiple outputs. We show that confidence estimated in this way correlates with image quality measured in terms of LPIPS values calculated for the generated and ground truth stained images. We validate our method by performing digital staining of images captured with a Differential Inference Contrast microscope on a dataset composed of white blood cells of 24 patients. The high absolute value of the correlation between our confidence score and LPIPS demonstrates the effectiveness of our method, opening the possibility of predicting the quality of generated output and ensuring trustworthiness in medical safety-critical setup.
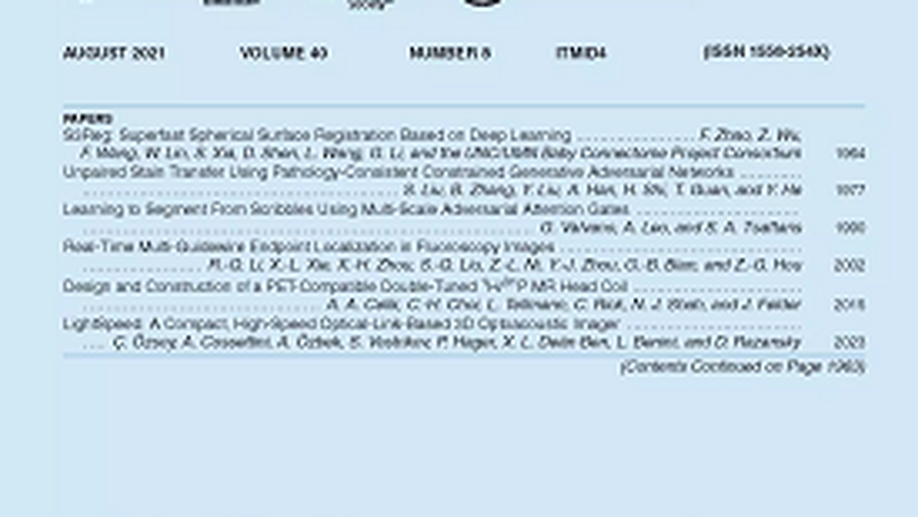
Multi-task multi-domain learning for digital staining and classification of leukocytes
oking stained images preserving the inter-cellular structures, crucial for the medical experts to perform classification. We achieve better structure preservation by adding auxiliary tasks of segmentation and direct reconstruction. Segmentation enforces that the network learns to generate correct nucleus and cytoplasm shape, while direct reconstruction enforces reliable translation between the matching images across domains. Besides, we build a robust domain agnostic latent space by injecting the target domain label directly to the generator, i.e., bypassing the encoder. It allows the encoder to extract features independently of the target domain and enables an automated domain invariant classification of the white blood cells. We validated our method on a large dataset composed of leukocytes of 24 patients, achieving state-of-the-art performance on both digital staining and classification tasks.
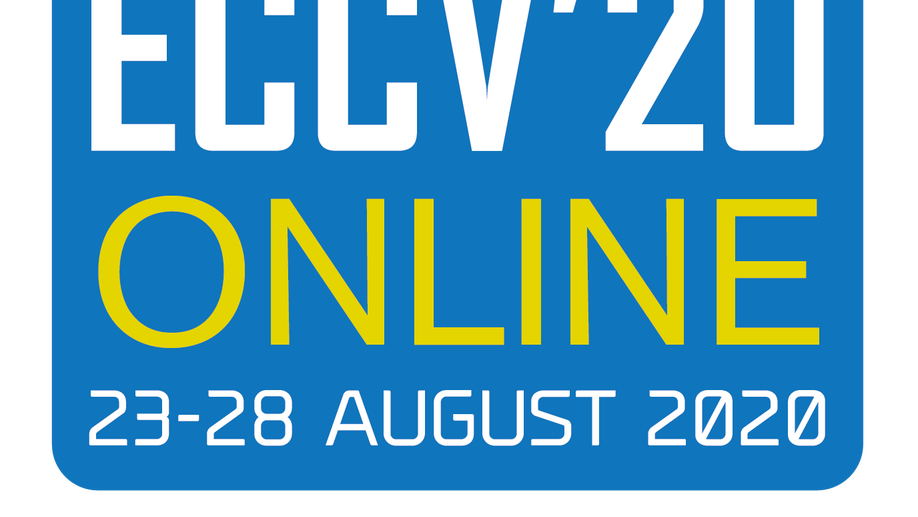
Fairness by Learning Orthogonal Disentangled Representations
Learning discriminative powerful representations is a crucial step for machine learning systems. Introducing invariance against arbitrary nuisance or sensitive attributes while performing well on specific tasks is an important problem in representation learning. This is mostly approached by purging the sensitive information from learned representations. In this paper, we propose a novel disentanglement approach to invariant representation problem. We disentangle the meaningful and sensitive representations by enforcing orthogonality constraints as a proxy for independence. We explicitly enforce the meaningful representation to be agnostic to sensitive information by entropy maximization. The proposed approach is evaluated on five publicly available datasets and compared with state of the art methods for learning fairness and invariance achieving the state of the art performance on three datasets and comparable performance on the rest. Further, we perform an ablative study to evaluate the effect of each component.
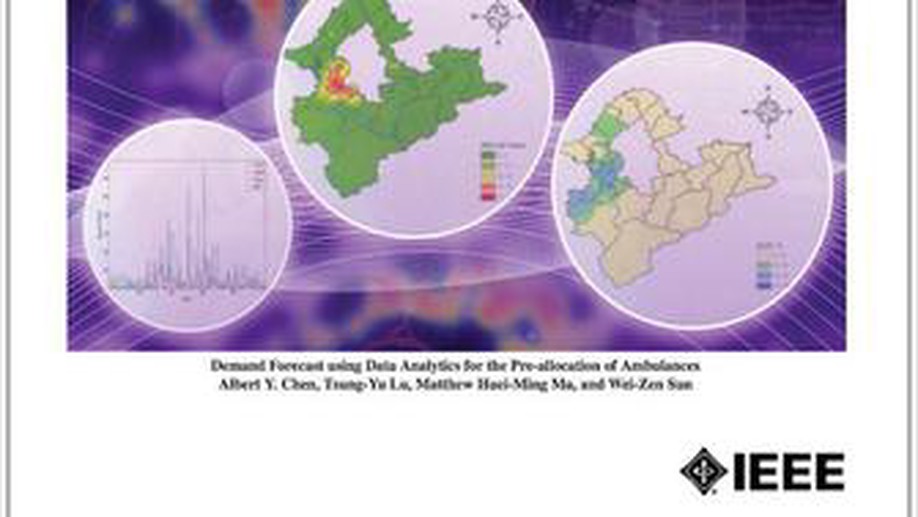
Seamless Virtual Whole Slide Image Synthesis and Validation Using Perceptual Embedding Consistency
Stain virtualization is an application with growing interest in digital pathology allowing simulation of stained tissue images thus saving lab and tissue resources. Thanks to the success of Generative Adversarial Networks (GANs) and the progress of unsupervised learning, unsupervised style transfer GANs have been successfully used to generate realistic, clinically meaningful and interpretable images. The large size of high resolution Whole Slide Images (WSIs) presents an additional computational challenge. This makes tilewise processing necessary during training and inference of deep learning networks. Instance normalization has a substantial positive effect in style transfer GAN applications but with tilewise inference, it has the tendency to cause a tiling artifact in reconstructed WSIs. In this paper we propose a novel perceptual embedding consistency (PEC) loss forcing the network to learn color, contrast and brightness invariant features in the latent space and hence substantially reducing the aforementioned tiling artifact. Our approach results in more seamless reconstruction of the virtual WSIs. We validate our method quantitatively by comparing the virtually generated images to their corresponding consecutive real stained images.We compare our results to state-of-the-art unsupervised style transfer methods and to the measures obtained from consecutive real stained tissue slide images. We demonstrate our hypothesis about the effect of the PEC loss by comparing model robustness to color, contrast and brightness perturbations and visualizing bottleneck embeddings. We validate the robustness of the bottleneck feature maps by measuring their sensitivity to the different perturbations and using them in a tumor segmentation task. Additionally, we propose a preliminary validation of the virtual staining application by comparing interpretation of 2 pathologists on real and virtual tiles and inter-pathologist agreement
Perceptual Embedding Consistency for Seamless Reconstruction of Tilewise Style Transfer
Style transfer is a field with growing interest and use cases in deep learning. Recent work has shown Generative Adversarial Networks(GANs) can be used to create realistic images of virtually stained slide images in digital pathology with clinically validated interpretability. Digital pathology images are typically of extremely high resolution, making tilewise analysis necessary for deep learning applications. It has been shown that image generators with instance normalization can cause a tiling artifact when a large image is reconstructed from the tilewise analysis. We introduce a novel perceptual embedding consistency loss significantly reducing the tiling artifact created in the reconstructed whole slide image (WSI). We validate our results by comparing virtually stained slide images with consecutive real stained tissue slide images. We also demonstrate that our model is more robust to contrast, color and brightness perturbations by running comparative sensitivity analysis tests.

Staingan: Stain style transfer for digital histological images
Digitized Histological diagnosis is in increasing demand. However, color variations due to various factors are imposing obstacles to the diagnosis process. The problem of stain color variations is a well-defined problem with many proposed solutions. Most of these solutions are highly dependent on a reference template slide. We propose a deep-learning solution inspired by cycle consistency that is trained end-to-end, eliminating the need for an expert to pick a representative reference slide. Our approach showed superior results quantitatively and qualitatively against the state of the art methods. We further validated our method on a clinical use-case, namely Breast Cancer tumor classification, showing 16% increase in AUC
Virtualization of tissue staining in digital pathology using an unsupervised deep learning approach
Histopathological evaluation of tissue samples is a key practice in patient diagnosis and drug development, especially in oncology. Historically, Hematoxylin and Eosin (H&E) has been used by pathologists as a gold standard staining. However, in many cases, various target specific stains, including immunohistochemistry (IHC), are needed in order to highlight specific structures in the tissue. As tissue is scarce and staining procedures are tedious, it would be beneficial to generate images of stained tissue virtually. Virtual staining could also generate in-silico multiplexing of different stains on the same tissue segment. In this paper, we present a sample application that generates FAP-CK virtual IHC images from Ki67-CD8 real IHC images using an unsupervised deep learning approach based on CycleGAN. We also propose a method to deal with tiling artifacts caused by normalization layers and we validate our approach by comparing the results of tissue analysis algorithms for virtual and real images.
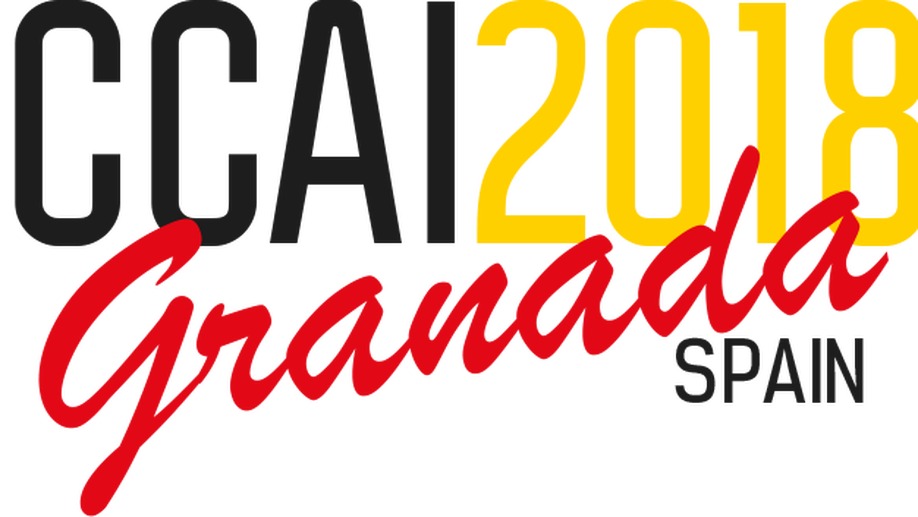
Domain and geometry agnostic CNNs for left atrium segmentation in 3D ultrasound
Segmentation of the left atrium and deriving its size can help to predict and detect various cardiovascular conditions. Automation of this process in 3D Ultrasound image data is desirable, since manual delineations are time-consuming, challenging and observer-dependent. Convolutional neural networks have made improvements in computer vision and in medical image analysis. They have successfully been applied to segmentation tasks and were extended to work on volumetric data. In this paper we introduce a combined deep-learning based approach on volumetric segmentation in Ultrasound acquisitions with incorporation of prior knowledge about left atrial shape and imaging device. The results show, that including a shape prior helps the domain adaptation and the accuracy of segmentation is further increased with adversarial learning.
Generalizing multistain immunohistochemistry tissue segmentation using one-shot color deconvolution deep neural networks
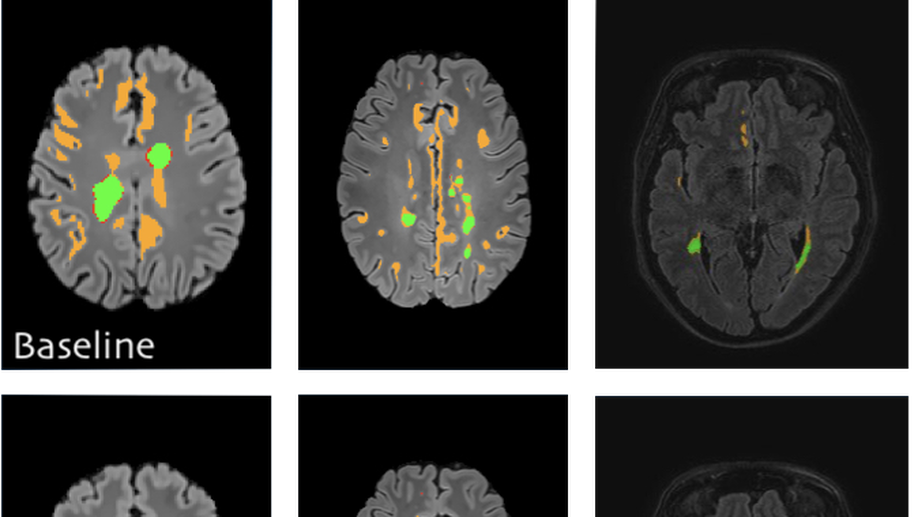
Semi-supervised deep learning for fully convolutional networks
Deep learning usually requires large amounts of labeled training data, but annotating data is costly and tedious. The framework of semi-supervised learning provides the means to use both labeled data and arbitrary amounts of unlabeled data for training. Recently, semi-supervised deep learning has been intensively studied for standard CNN architectures. However, Fully Convolutional Networks (FCNs) set the state-of-the-art for many image segmentation tasks. To the best of our knowledge, there is no existing semi-supervised learning method for such FCNs yet. We lift the concept of auxiliary manifold embedding for semi-supervised learning to FCNs with the help of Random Feature Embedding. In our experiments on the challenging task of MS Lesion Segmentation, we leverage the proposed framework for the purpose of domain adaptation and report substantial improvements over the baseline model.