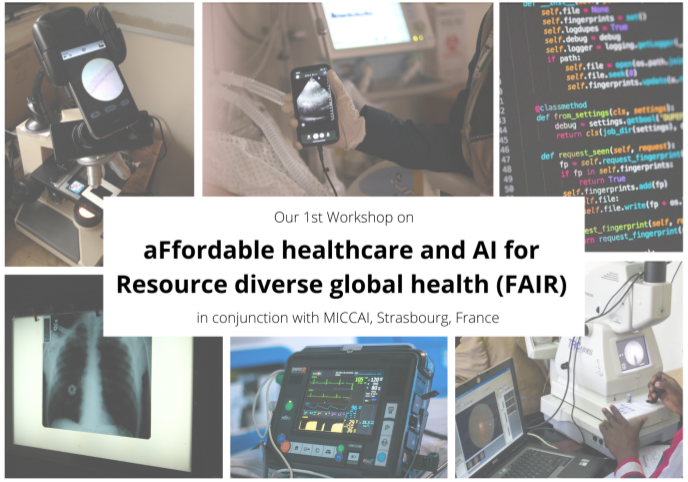
We are also interested in developing affordable AI solutions suitable for poor-quality data generated by low infrastructure and point-of-care diagnosis.
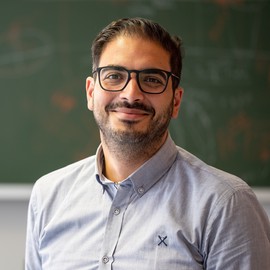
Shadi Albarqouni
Professor of Computational Medical Imaging Research at University of Bonn | AI Young Investigator Group Leader at Helmholtz AI | Affiliate Scientist at Technical University of Munich
Related
Publications
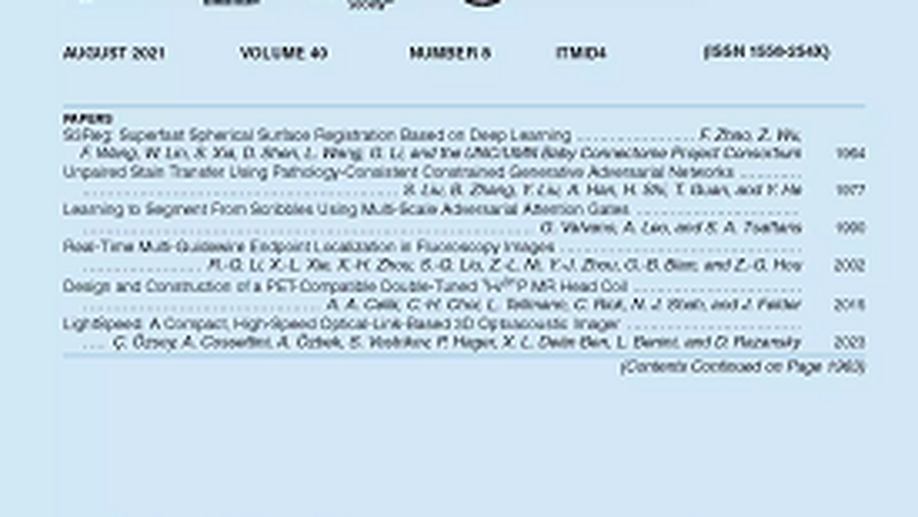
Digital staining of white blood cells with confidence estimation
Chemical staining of the blood smears is one of the crucial components of blood analysis. It is an expensive, lengthy and sensitive process, often prone to produce slight variations in colour and seen structures due to a lack of unified protocols across laboratories. Even though the current developments in deep generative modeling offer an opportunity to replace the chemical process with a digital one, there are specific safety-ensuring requirements due to the severe consequences of mistakes in a medical setting. Therefore digital staining system would profit from an additional confidence estimation quantifying the quality of the digitally stained white blood cell. To this aim, during the staining generation, we disentangle the latent space of the Generative Adversarial Network, obtaining separate representation s of the white blood cell and the staining. We estimate the generated image’s confidence of white blood cell structure and staining quality by corrupting these representations with noise and quantifying the information retained between multiple outputs. We show that confidence estimated in this way correlates with image quality measured in terms of LPIPS values calculated for the generated and ground truth stained images. We validate our method by performing digital staining of images captured with a Differential Inference Contrast microscope on a dataset composed of white blood cells of 24 patients. The high absolute value of the correlation between our confidence score and LPIPS demonstrates the effectiveness of our method, opening the possibility of predicting the quality of generated output and ensuring trustworthiness in medical safety-critical setup.
Fourier Transform of Percoll Gradients Boosts CNN Classification of Hereditary Hemolytic Anemias
Sickle Cell Disease Severity Prediction from Percoll Gradient Images using Graph Convolutional Networks

Staingan: Stain style transfer for digital histological images
Digitized Histological diagnosis is in increasing demand. However, color variations due to various factors are imposing obstacles to the diagnosis process. The problem of stain color variations is a well-defined problem with many proposed solutions. Most of these solutions are highly dependent on a reference template slide. We propose a deep-learning solution inspired by cycle consistency that is trained end-to-end, eliminating the need for an expert to pick a representative reference slide. Our approach showed superior results quantitatively and qualitatively against the state of the art methods. We further validated our method on a clinical use-case, namely Breast Cancer tumor classification, showing 16% increase in AUC