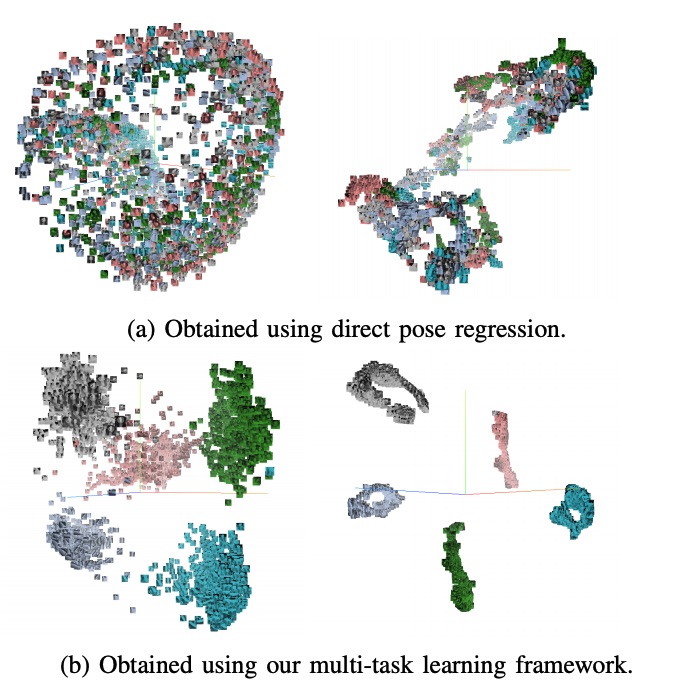
Together with our clinical and industry partners, we realized that there is a need to incorporate domain-specific knowledge and let the model Learn from a Prior Knowledge. We first investigated modeling general priors, i.e., manifold assumptions, to learn powerful representations. Such representations achieved state-of-the-art on benchmark datasets, such as e IDRiD for Diabetic Retinopathy Early Detection (Sarhan et al. 2019), and 7 Scenes for Camera Relocalization (Bui et al. 2017). Then, we started looking into the laplacian graph, where prior knowledge can be modeled as a soft constraint, i.e., regularization, to learn feature representation that follows such manifold defined by graphs. We have shown in our ISBI (Kazi et al. 2019a), MICCAI (Kazi et al. 2019b), and IPMI (Kazi et al. 2019) papers that leveraging prior knowledge such as proximity of ages, gender, and a few lab results, are of high importance in Alzheimer classification.
Collaboration:
Funding:
- Siemens AG
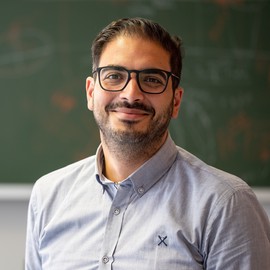
Shadi Albarqouni
Professor of Computational Medical Imaging Research at University of Bonn | AI Young Investigator Group Leader at Helmholtz AI | Affiliate Scientist at Technical University of Munich
Related
Publications
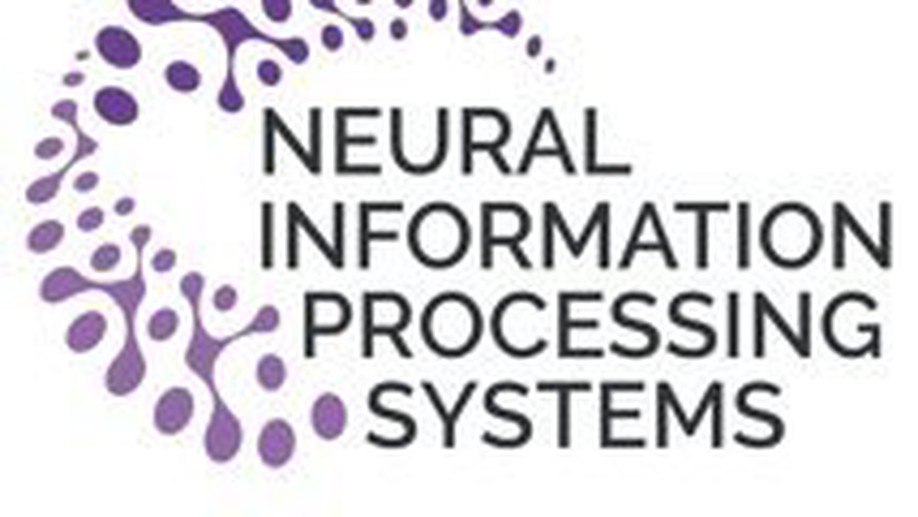
Lvm-med: Learning large-scale self-supervised vision models for medical imaging via second-order graph matching
Obtaining large pre-trained models that can be fine-tuned to new tasks with limited annotated samples has remained an open challenge for medical imaging data. While pre-trained deep networks on ImageNet and vision-language foundation models trained on web-scale data are prevailing approaches, their effectiveness on medical tasks is limited due to the significant domain shift between natural and medical images. To bridge this gap, we introduce LVM-Med, the first family of deep networks trained on large-scale medical datasets. We have collected approximately 1.3 million in medical images from 55 publicly available datasets, covering a large number of organs and modalities such as CT, MRI, X-ray, and Ultrasound. We benchmark several state-of-the-art self-supervised algorithms on this dataset and propose a novel self-supervised contrastive learning algorithm using a graph matching formulation. The proposed approach makes three contributions: (i) it integrates prior pair-wise image similarity metrics based on local and global information; (ii) it captures the structural constraints of feature embeddings through a loss function constructed via a combinatorial graph-matching objective; and (iii) it can be trained efficiently end-to-end using modern gradient-estimation techniques for black-box solvers. We thoroughly evaluate the proposed LVM-Med on 15 downstream medical tasks ranging from segmentation and classification to object detection, and both for the in and out-of-distribution settings. LVM-Med empirically outperforms a number of state-of-the-art supervised, self-supervised, and foundation models. For challenging tasks such as Brain Tumor Classification or Diabetic Retinopathy Grading, LVM-Med improves previous vision-language models trained on 1 billion masks by 6-7% while using only a ResNet-50. We release pre-trained models at this link https://github.com/duyhominhnguyen/LVM-Med.
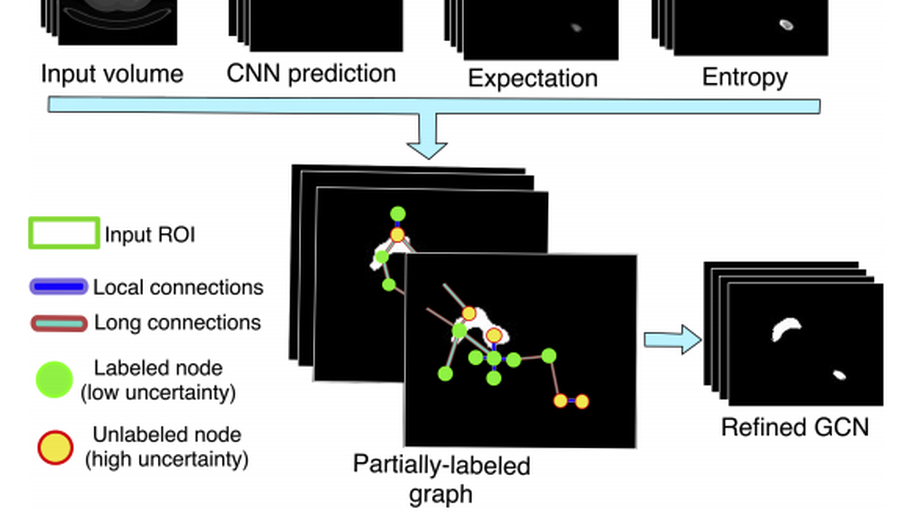
An Uncertainty-Driven GCN Refinement Strategy for Organ Segmentation
Organ segmentation in CT volumes is an important pre-processing step in many computer assisted intervention and diagnosis methods. In recent years, convolutional neural networks have dominated the state of the art in this task. However, since this problem presents a challenging environment due to high variability in the organ’s shape and similarity between tissues, the generation of false negative and false positive regions in the output segmentation is a common issue. Recent works have shown that the uncertainty analysis of the model can provide us with useful information about potential errors in the segmentation. In this context, we proposed a segmentation refinement method based on uncertainty analysis and graph convolutional networks. We employ the uncertainty levels of the convolutional network in a particular input volume to formulate a semi-supervised graph learning problem that is solved by training a graph convolutional network. To test our method we refine the initial output of a 2D U-Net. We validate our framework with the NIH pancreas dataset and the spleen dataset of the medical segmentation decathlon. We show that our method outperforms the state-of-the-art CRF refinement method by improving the dice score by 1% for the pancreas and 2% for spleen, with respect to the original U-Net’s prediction. Finally, we perform a sensitivity analysis on the parameters of our proposal and discuss the applicability to other CNN architectures, the results, and current limitations of the model for future work in this research direction. For reproducibility purposes, we make our code publicly available at https://github.com/rodsom22/gcn_refinement
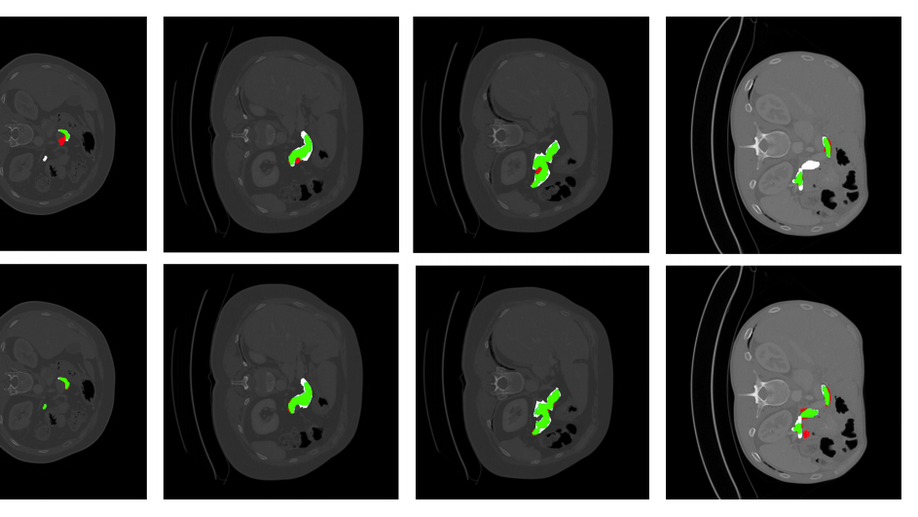
Uncertainty-based graph convolutional networks for organ segmentation refinement
Organ segmentation is an important pre-processing step in many computer assisted intervention and diagnosis methods. In recent years, CNNs have dominated the state of the art in this task. Organ segmentation scenarios present a challenging environment for these methods due to high variability in shape and similarity with background. This leads to the generation of false negative and false positive regions in the output segmentation. In this context, the uncertainty analysis of the model can provide us with useful information about potentially misclassified elements. In this work we propose a method based on uncertainty analysis and graph convolutional networks as a post-processing step for segmentation. For this, we employ the uncertainty levels of the CNN to formulate a semi-supervised graph learning problem that is solved by training a GCN on the low uncertainty elements. Finally, we evaluate the full graph on the trained GCN to get the refined segmentation. We test our framework in refining the output of pancreas and spleen segmentation models. We show that the framework can increase the average dice score in 1% and 2% respectively for these problems. Finally, we discuss the results and current limitations of the model that lead to future work in this research direction
Adaptive image-feature learning for disease classification using inductive graph networks
Recently, Geometric Deep Learning (GDL) has been introduced as a novel and versatile framework for computer-aided disease classification. GDL uses patient meta-information such as age and gender to model patient cohort relations in a graph structure. Concepts from graph signal processing are leveraged to learn the optimal mapping of multi-modal features, e.g. from images to disease classes. Related studies so far have considered image features that are extracted in a pre-processing step. We hypothesize that such an approach prevents the network from optimizing feature representations towards achieving the best performance in the graph network. We propose a new network architecture that exploits an inductive end-to-end learning approach for disease classification, where filters from both the CNN and the graph are trained jointly. We validate this architecture against state-of-the-art inductive graph networks and demonstrate significantly improved classification scores on a modified MNIST toy dataset, as well as comparable classification results with higher stability on a chest X-ray image dataset. Additionally, we explain how the structural information of the graph affects both the image filters and the feature learning.
Graph Convolution Based Attention Model for Personalized Disease Prediction
Clinicians implicitly incorporate the complementarity of multi-modal data for disease diagnosis. Often a varied order of importance for this heterogeneous data is considered for personalized decisions. Current learning-based methods have achieved better performance with uniform attention to individual information, but a very few have focused on patient-specific attention learning schemes for each modality. Towards this, we introduce a model which not only improves the disease prediction but also focuses on learning patient-specific order of importance for multi-modal data elements. In order to achieve this, we take advantage of LSTM-based attention mechanism and graph convolutional networks (GCNs) to design our model. GCNs learn multi-modal but class-specific features from the entire population of patients, whereas the attention mechanism optimally fuses these multi-modal features into a final decision, separately for each patient. In this paper, we apply the proposed approach for disease prediction task for Parkinson’s and Alzheimer’s using two public medical datasets.
InceptionGCN: receptive field aware graph convolutional network for disease prediction
Geometric deep learning provides a principled and versatile manner for the integration of imaging and non-imaging modalities in the medical domain. Graph Convolutional Networks (GCNs) in particular have been explored on a wide variety of problems such as disease prediction, segmentation, and matrix completion by leveraging large, multimodal datasets. In this paper, we introduce a new spectral domain architecture for deep learning on graphs for disease prediction. The novelty lies in defining geometric ‘inception modules’ which are capable of capturing intra- and inter-graph structural heterogeneity during convolutions. We design filters with different kernel sizes to build our architecture. We show our disease prediction results on two publicly available datasets. Further, we provide insights on the behaviour of regular GCNs and our proposed model under varying input scenarios on simulated data.
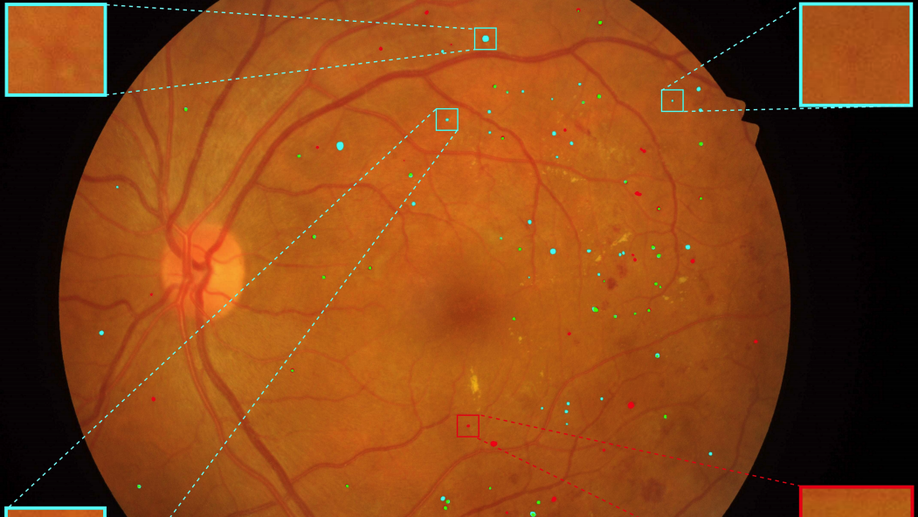
Multi-scale Microaneurysms Segmentation Using Embedding Triplet Loss
Deep learning techniques are recently being used in fundus image analysis and diabetic retinopathy detection. Microaneurysms are an important indicator of diabetic retinopathy progression. We introduce a two-stage deep learning approach for microaneurysms segmentation using multiple scales of the input with selective sampling and embedding triplet loss. The model first segments on two scales and then the segmentations are refined with a classification model. To enhance the discriminative power of the classification model, we incorporate triplet embedding loss with a selective sampling routine. The model is evaluated quantitatively to assess the segmentation performance and qualitatively to analyze the model predictions. This approach introduces a 30.29% relative improvement over the fully convolutional neural network.
Self-attention equipped graph convolutions for disease prediction
Multi-modal data comprising imaging (MRI, fMRI, PET, etc.) and non-imaging (clinical test, demographics, etc.) data can be collected together and used for disease prediction. Such diverse data gives complementary information about the patient’s condition to make an informed diagnosis. A model capable of leveraging the individuality of each multi-modal data is required for better disease prediction. We propose a graph convolution based deep model which takes into account the distinctiveness of each element of the multi-modal data. We incorporate a novel self-attention layer, which weights every element of the demographic data by exploring its relation to the underlying disease. We demonstrate the superiority of our developed technique in terms of computational speed and performance when compared to state-of-the-art methods. Our method outperforms other methods with a significant margin.
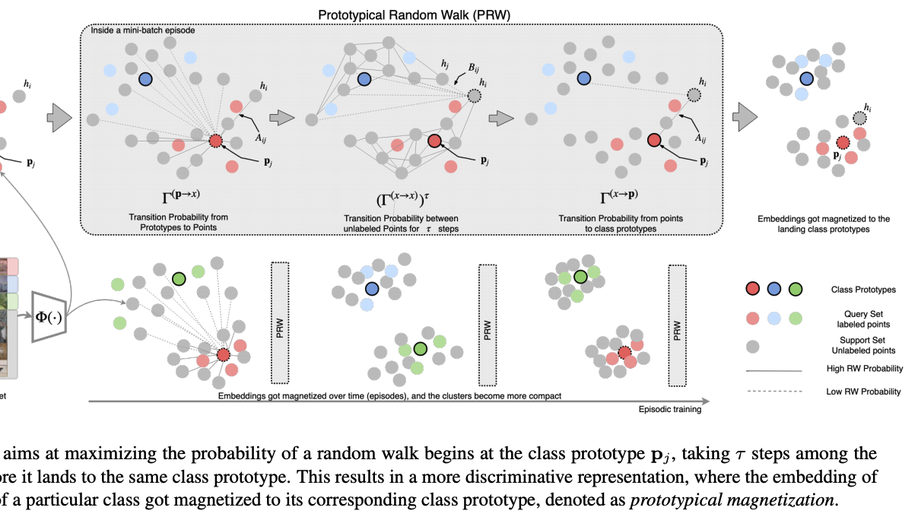
Semi-Supervised Few-Shot Learning with Prototypical Random Walks
Recent progress has shown that few-shot learning can be improved with access to unlabelled data, known as semi-supervised few-shot learning(SS-FSL). We introduce an SS-FSL approach, dubbed as Prototypical Random Walk Networks(PRWN), built on top of Prototypical Networks (PN). We develop a random walk semi-supervised loss that enables the network to learn representations that are compact and well-separated. Our work is related to the very recent development on graph-based approaches for few-shot learning. However, we show that compact and well-separated class representations can be achieved by modeling our prototypical random walk notion without needing additional graph-NN parameters or requiring a transductive setting where collective test set is provided. Our model outperforms prior art in most benchmarks with significant improvements in some cases. For example, in a mini-Imagenet 5-shot classification task, we obtain 69.65% accuracy to the 64.59% state-of-the-art. Our model, trained with 40% of the data as labelled, compares competitively against fully supervised prototypical networks, trained on 100% of the labels, even outperforming it in the 1-shot mini-Imagenet case with 50.89% to 49.4% accuracy. We also show that our model is resistant to distractors, unlabeled data that does not belong to any of the training classes, and hence reflecting robustness to labelled/unlabelled class distribution mismatch. We also performed a challenging discriminative power test, showing a relative improvement on top of the baseline of ≈14% on 20 classes on mini-Imagenet and ≈60% on 800 classes on Omniglot. Code will be made available.
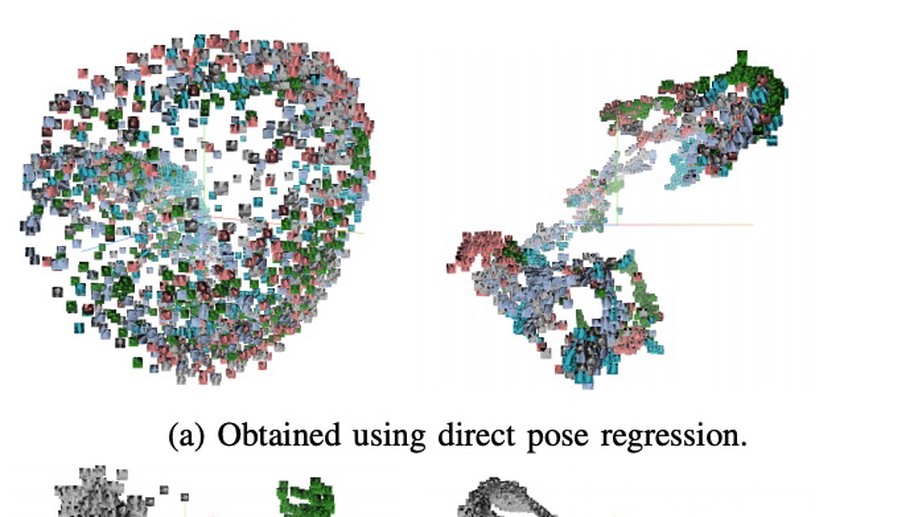
When regression meets manifold learning for object recognition and pose estimation
In this work, we propose a method for object recognition and pose estimation from depth images using convolutional neural networks. Previous methods addressing this problem rely on manifold learning to learn low dimensional viewpoint descriptors and employ them in a nearest neighbor search on an estimated descriptor space. In comparison we create an efficient multi-task learning framework combining manifold descriptor learning and pose regression. By combining the strengths of manifold learning using triplet loss and pose regression, we could either estimate the pose directly reducing the complexity compared to NN search, or use learned descriptor for the NN descriptor matching. By in depth experimental evaluation of the novel loss function we observed that the view descriptors learned by the network are much more discriminative resulting in almost 30% increase regarding relative pose accuracy compared to related works. On the other hand, regarding directly regressed poses we obtained important improvement compared to simple pose regression. By leveraging the advantages of both manifold learning and regression tasks, we are able to improve the current state-of-the-art for object recognition and pose retrieval that we demonstrate through in depth experimental evaluation.
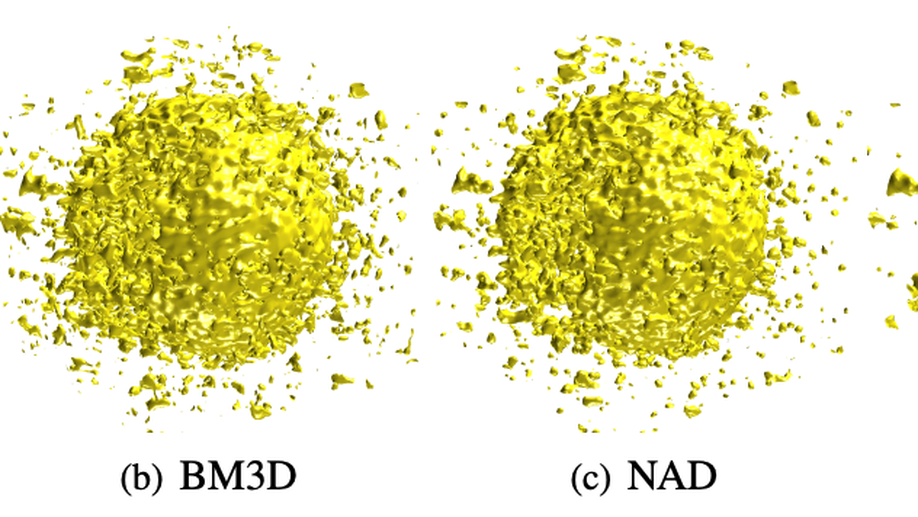