Learn to Learn
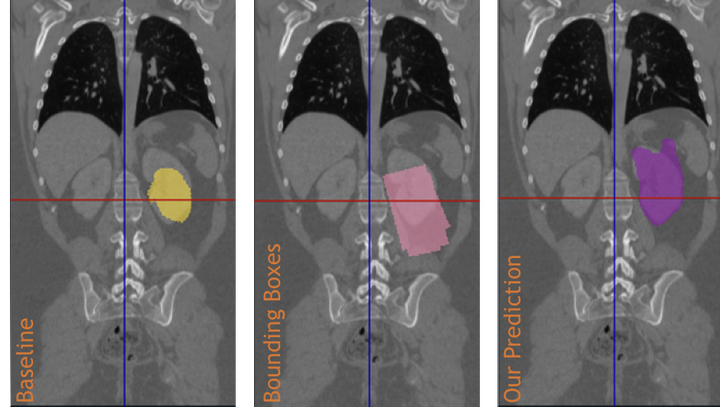
To build models that are transferable to different tasks or different data distributions, i.e., non i.i.d., we have investigated meta-learning approaches such as prototypical networks (PN) (Snell et al. 2017). PN learns a class prototype from very few amounts of labeled data, e.g., 1-5 shots, and use the learned prototypes to perform the classification tasks. In the context of medical imaging, we were first to introduce Few-Shot Learning into the MIC community. We have shown in our recent ICML Workshop paper (Ayyad et al. 2019) that our novel Semi-Supervised Few-Shot Learning achieves the state-of-the-art on benchmark datasets; Omniglot, miniImageNet, and TieredImageNet. Further, we have demonstrated in our recent paper (Parida et al. 2019) that such concepts can be utilized in medical imaging segmentation with an extremely low budget of annotated data, e.g., bounding boxes, and better generalization capability, i.e., to new organs or anomalies, however, at the cost of less accurate segmentation. Yet, our proposed models have great potential in clinical practice where a novel application could come in, and only a very few annotations are required, to perform segmentation tasks. Further, such a learning paradigm has a great potential in Federated Learning, where the data acquired at different hospitals capture heterogeneous and non i.i.d data, i.e., various tasks, making proposed models suitable for such a problem.