Learn to Reason and Explain
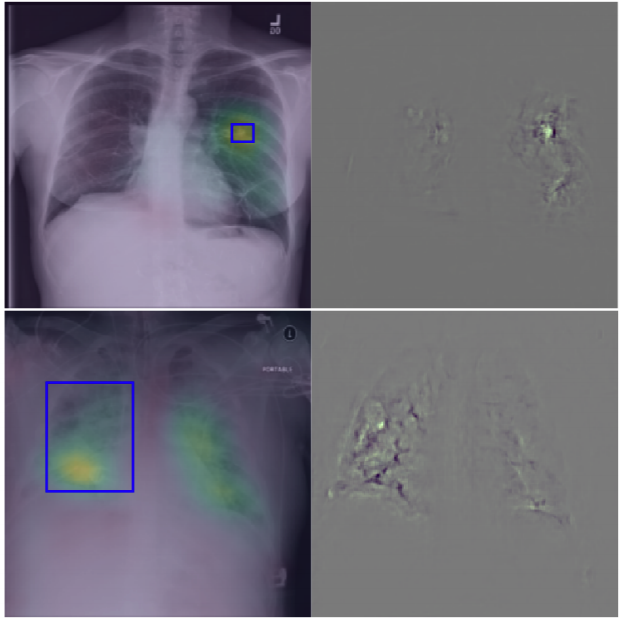
To build explainable AI models that are interpretable for our end-users, i.e., clinicians, we have investigated two research directions. First, we have utilized some visualization techniques to explain and interpret “black box” models by propagating back the gradient of the class of interest to the image space where you can see the relevant semantics, so-called Gradient Class Activation Maps (GradCAM). Sooner, we found out such techniques do not produce meaningful results. In other words, irrelevant semantics could be highly activated in GradCAM, yielding unreliable explanation tools. To overcome such a problem, we have introduced a robust optimization loss in our MICCAI paper (Khakzar et al. 2019), which generated adversarial examples enforcing the network to only focus on relevant features and probably correlated with other examples belonging to the same class.
Second, we have investigated designing and building explainable models by i) uncertainty quantification and ii) disentangled feature representation. In the first category, we started understanding the uncertainty estimates generated by Monte-Carlo Dropout, the approximate of Bayesian Neural Networks, and other techniques, e.g. PointNet, in Camera Relocalization problem (Bui et al. 2018), to shed light on the ambiguity present in the dataset. We took a step further, and use such uncertainty estimates to refine the segmentation in an unsupervised fashion (Soberanis-Mukul et al. 2019, Bui et al. 2019).
Recently, we have investigated modeling the labels uncertainty, which is related to the inter-/intra-observer variability, and produced a metric to quantify such uncertainty. We have shown in our paper (Tomczack et al. 2019) that such uncertainty can be rather disentangled from the model and data uncertainties, so-called, epistemic, and aleatoric uncertainties, respectively. We believe such uncertainty is of high importance to the referral systems. In the second category, we have studied the variational methods, and disentangled representations, where the assumption here that some generative factors, e.g., color, shape, and pathology, will be captured in the lower-dimensional latent space, and one can easily go through the manifold and generate tons of example by sampling from the posterior distribution. We were among the firsts who introduce such concepts in medical imaging by investigating the influence of residual blocks and adversarial learning on disentangled representation (Sarhan et al. 2019). Our hypothesis that better reconstruction fidelity would force the network to model high resolution, which might have a positive influence on the disentangled representation, in particular, some pathologies.
Collaboration:
- Dr. Abouzar Eslami, Carl Zeiss Meditec AG
- PD. Dr. Slobodan Ilic, Siemens AG
Funding:
- Siemens AG